Research Article | Open Access
Prediction of Metastatic Site Based On Somatic Gene Mutations in Primary Tumors in Prostate Cancer
Paul Gomez11Nanobiotek, LLC, Florida, USA.
Correspondence: Paul Gomez (Nanobiotek, LLC, 1541 Riverside Ave, Jacksonville, Florida 32204, USA; Email: pgomez@nano-bio-tek.com).
Annals of Urologic Oncology 2023, 6(4): 19-27. https://doi.org/10.32948/auo.2023.12.22
Received: 28 Nov 2023 | Accepted: 19 Dec 2023 | Published online: 24 Dec 2023
Background The most common metastatic targets in prostate cancer (PCa) are the pelvic lymph nodes (PLN) and bones. The PLNs are routinely dissected by a procedure called pelvic lymph node dissection (PLND) simultaneously with the surgical removal of the prostate to detect the presence of metastatic growths. Additionally, the prostate-specific antigen (PSA) level is used to assess the existence of a metastatic stage. However, micrometastases in other organs and tissues might be overlooked.
Methods We downloaded publicly available prostate cancer tumor data from the website www.CbioPortal.org. After choosing the 25 most frequently mutated genes by metastatic site (MS) and finding genes that are uniquely mutated on specific metastatic sites, we found that the mutational signature of a prostate cancer tumor is associated with its MS, and thus, we developed a method to numerically predict this association.
Results After executing a computational algorithm on the data set of metastatic prostate tumors, it was found that we can predict metastatic sites with the following accuracies: bone (90.9%), retroperitoneum (87.5%), liver (83.0%), kidney (80.0%), pancreas (80.0%), adrenal glands (75.0%), lung (71.1%), and brain (72.5%).
Conclusions We successfully developed a method and an algorithm that predict the most likely metastatic site of a primary prostate cancer tumor based on its genetic mutations. The accuracy of the predictions for eight metastatic sites ranges from 71.1% to 90.9%, with an average of 80.5%.
Key words cancer, prostate cancer, metastasis, carcinoma, adenocarcinoma, data science
Besides the LN, PCa metastasizes preferentially to the bones [8-10]. Several imaging techniques exist to visualize bone metastases, namely, Whole-Body magnetic resonance imaging (WB-MRI) [11], positron emission tomography/computer tomography (PET/CT) [12], and others. With WB-MRI, a standard protocol has been established that targets the 14 most frequent metastatic sites (MS) identified on the Metastasis Reporting and Data System for Prostate Cancer (MET-RADS-P) template [13] shown on Figure 1.
It should be noted that the brain and other less frequent metastatic sites, such as the spleen, pancreas, and the adrenal glands, are not included on the template. They all fall under the category “Other sites”. Those less frequent MS cases combined are less than 5% of all cases in our study but correspond to 47 patients, who could probably be saved by tailoring the WB-MRI to image specific organs should our predicting tool indicates a high probability of metastasis to those sites.
However, in many instances, no metastatic lesions are found after performing a WB-MRI. The main reason is that, although there might be micrometastases growing, their sizes are so small that they cannot be detected at the time of imaging.
We developed a computational tool that predicts the existence of metastasis in specific organs based on the frequency of the mutations found in the primary tumor. Not finding clinical evidence of metastasis after RP should not mean that metastasis can be completely ruled out. Overlooking those MS could lead to unfavorable outcomes in the near future.
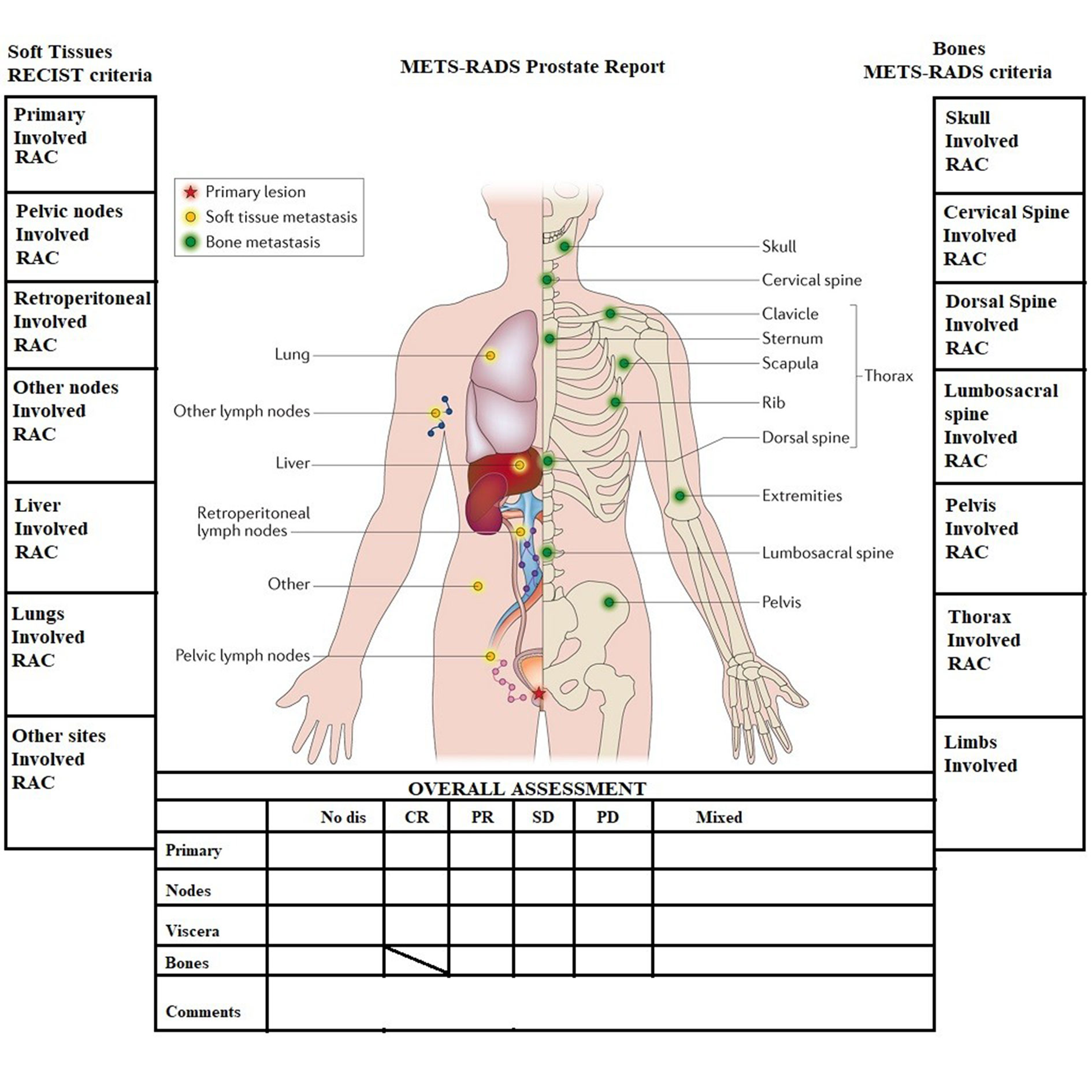
We downloaded prostate adenocarcinoma (PRAD) tumor data from the website www.CbioPortal.org [14], which holds cancer samples for all types of cancer. The data have been shared by several institutions and are publicly available. Using database methods, we cleaned up the data and selected all metastatic prostate cancer samples for detailed examination.
After choosing the 25 most frequently mutated genes by metastatic site and finding genes that are uniquely mutated on specific metastatic sites, we found that the mutational signature of a prostate cancer tumor is associated with its metastatic site, and thus, we developed a method to numerically predict this association. We obtained the frequency of mutations by gene and by metastatic site across the whole population of metastatic tumors.
The computer technology we used was Microsoft SQL Server 2022 to host the data and implement the prediction algorithm, and Python 3.10 (64-bit) to import the data into the SQL database.
We started with 1,758 metastatic prostate tumors, of which 636 metastasized to the lymph nodes. We excluded these tumors, as it was explained before. This study also excluded these other organs: the skin, muscles, penis, thorax, neck, urethra, and rectum, due to the small number of cases (<=3).
Additionally, the spleen (n=10) and the bladder (n=7) had to be excluded due to poor prediction accuracy (20% and 42%, respectively).
The final list of 8 metastatic sites we worked with for predicting the most likely MS is shown on Table 1, and graphically on the pie chart of Figure 2.
A total of 847 metastatic tumors were used for further analysis. It can be seen from Table 1 and Figure 2 that the number of cases in which PCa metastasizes to the brain, kidney, adrenal glands, retroperitoneum, and pancreas is very small. However, when we analyzed the most commonly mutated genes by MS, it was discovered that they vary substantially depending on the metastatic site. This fact allowed us to design an algorithm based on competition.
The first step in the analysis of somatic mutations in PRAD neoplasms was to obtain the frequency of mutations in the top 25 most mutated genes. The rate of mutations was split into primary and metastatic genes, and then a list from an external source [15] was used for comparison. The results of this analysis are shown in Table 2.
The most commonly mutated genes are: TP53, SPOP, FOXA1, AR, PTEN, TTN, KMT2C, KMT2D, BRCA2, and others. On this list, TP53 is found to be the most highly mutated gene. It is known that TP53 has the most frequent alterations in all cancer types [16-18]. Being a common denominator, TP53 was excluded from this research.
The last set of mutations in Table 2 includes both primary and metastatic tumors. An important gene, Androgen Receptor (AR), appears highly mutated on this set and in our metastatic tumors list, but is not highly ranked in our primary tumors list. This is consistent with the fact that AR mutations are usually found to increase with cancer stage [19]. The androgen receptor (AR) has been used as the target for androgen deprivation therapy to treat de novo or recurrent metastatic disease [20]. Although this therapy works for a while, inhibiting tumor growth, most patients eventually develop resistance, and the disease becomes castration-resistant prostate cancer. AR is mutated at different rates at different metastatic sites, and thus, it was included in the calculations and algorithm.
The 25 most highly mutated genes by metastatic site were obtained, resulting in a total of 124 different genes. This indicates the wide variety of most-ranked mutated genes across the 8 metastatic sites analyzed in this research. Genes that are not mutated in at least 3 tumors were excluded, resulting in some metastatic sites having fewer than 25 most frequently mutated genes.
We define the “rank” as the order in which a gene appears most frequently mutated, #1 being the highest mutated and #25 the least mutated.
In Table 3, it can be seen that gene FOXA1 has the highest rank (#1) in metastases to the bone and lungs, but in metastases to the liver, it is ranked #9. FOXA1 does not show up as one of the most-ranked genes in other metastatic sites.
Bone and liver metastases have several common mutated genes, but at different rates of mutation. Bone and lung share less commonly mutated genes, and finally, bone metastases have no common mutated genes with the brain, kidney, and pancreas; only one common gene with retroperitoneum metastasis (APC); and only 3 common genes with adrenal gland metastases: TTN, MUC4, and ABCA13.
A similar analysis was done with all other metastatic sites, and it was found that the frequency of mutated genes among them is a feature that can be exploited as a pattern recognition variable. The lists of the top 25 most mutated genes in those 8 metastatic sites are greatly diverse.
Additionally, we found a list of 111 genes that are uniquely mutated at specific metastatic sites. These are genes that belong to the list of the top 25 most mutated genes. Metastases to the pancreas have the most number of unique mutated genes: 25. For this reason, even though we only have 5 cases of metastasis to the pancreas, it was possible to classify those tumors with good accuracy.
The Prediction Algorithm
Based on our previous analysis, we designed an algorithm that, given a list of somatic mutations in a prostate cancer tumor, will predict the three most likely metastatic sites in order of probability.
The algorithm has 2 main steps:
(1) Determine if the tumor has 4 or more genes that belong to the list of unique genes for a specific site. If that is the case, the metastatic site is predicted, and no further analysis is done.
(2) If the metastatic site cannot be predicted by the uniqueness of its genes, a list of 8 scores, one per metastatic site, is calculated. For each gene in the tumor, the score of each metastatic site is calculated as the sum of the multiplication of each gene’s percentage within the MS times the percentage of the MS. This is a competition algorithm based on the variability of the weights of metastatic sites and genes within its MS.
(3) At the end, the list is sorted by final score, and the three metastatic sites with the highest score are the answer.
Table 1. Metastatic Sites in Prostate Cancer excluding LN. |
||
Metastatic site |
Cases (n) |
Percentage |
Bone |
506 |
59.7% |
Liver |
218 |
25.7% |
Lung |
76 |
9.0% |
Brain |
16 |
1.9% |
Kidney |
10 |
1.2% |
Adrenal Gland |
8 |
0.9% |
Retroperitoneum |
8 |
0.9% |
Pancreas |
5 |
0.6% |
Table 2. Frequency of mutations of the top 25 most mutated genes in PRAD. |
||||||||||
Primary Tumors |
Metastatic Tumors |
External Source [15], All Tumors |
||||||||
Gene |
Tumors |
PCT (%) |
|
Gene |
Tumors |
PCT (%) |
|
Gene |
Tumors |
PCT (%) |
TP53 |
364 |
13.5 |
|
TP53 |
766 |
16.0 |
|
TP53 |
455 |
16.1 |
SPOP |
292 |
10.8 |
|
TTN |
348 |
7.3 |
|
FOXA1 |
218 |
7.7 |
FOXA1 |
222 |
8.2 |
|
AR |
270 |
5.6 |
|
SPOP |
214 |
7.6 |
TTN |
151 |
5.6 |
|
FOXA1 |
254 |
5.3 |
|
AR |
190 |
6.7 |
KMT2C |
150 |
5.6 |
|
MUC16 |
235 |
4.9 |
|
KMT2C |
147 |
5.2 |
KMT2D |
135 |
5.0 |
|
KMT2C |
215 |
4.5 |
|
KMT2D |
132 |
4.7 |
ZFHX3 |
114 |
4.2 |
|
KMT2D |
205 |
4.3 |
|
CSMD3 |
115 |
4.1 |
PTEN |
107 |
4.0 |
|
CDK12 |
205 |
4.3 |
|
ATM |
86 |
3.1 |
CDK12 |
104 |
3.9 |
|
MUC4 |
193 |
4.0 |
|
APC |
84 |
3.0 |
MUC16 |
92 |
3.4 |
|
SYNE1 |
182 |
3.8 |
|
PTEN |
81 |
2.9 |
APC |
88 |
3.3 |
|
SPOP |
179 |
3.7 |
|
CDK12 |
74 |
2.6 |
ATM |
80 |
3.0 |
|
APC |
178 |
3.7 |
|
CTNNB1 |
72 |
2.6 |
PIK3CA |
75 |
2.8 |
|
PTEN |
171 |
3.6 |
|
LRP1B |
68 |
2.4 |
KDM6A |
74 |
2.7 |
|
ZFHX3 |
161 |
3.4 |
|
KDM6A |
54 |
1.9 |
SPEN |
71 |
2.6 |
|
CSMD3 |
132 |
2.8 |
|
ZFHX3 |
52 |
1.8 |
BRCA2 |
66 |
2.5 |
|
BRCA2 |
125 |
2.6 |
|
PIK3CA |
49 |
1.7 |
SYNE1 |
66 |
2.5 |
|
OBSCN |
121 |
2.5 |
|
BRCA2 |
46 |
1.6 |
KMT2A |
60 |
2.2 |
|
ATM |
115 |
2.4 |
|
MED12 |
42 |
1.5 |
MED12 |
59 |
2.2 |
|
DST |
110 |
2.3 |
|
ZMYM3 |
39 |
1.4 |
HMCN1 |
58 |
2.2 |
|
HMCN1 |
107 |
2.2 |
|
RB1 |
33 |
1.2 |
JAK1 |
57 |
2.1 |
|
MT-ND5 |
106 |
2.2 |
|
CTNNA2 |
27 |
1.0 |
FAT1 |
55 |
2.0 |
|
RYR2 |
106 |
2.2 |
|
GRIN2A |
23 |
0.8 |
PTPRD |
51 |
1.9 |
|
CTNNB1 |
106 |
2.2 |
|
AKT1 |
22 |
0.8 |
SPTA1 |
51 |
1.9 |
|
RB1 |
102 |
2.1 |
|
ARID1B |
19 |
0.7 |
MGA |
50 |
1.9 |
|
GRIN2A |
101 |
2.1 |
|
FAT4 |
19 |
0.7 |
Table 3. Mutational Landscape of Bone Metastasis vs. Other Metastatic Sites. |
||||||||||||||||
Bone |
Liver |
Lung |
Brain |
Kidney |
Adrenal gland |
Retroperiotoneum |
Pancreas |
|||||||||
GENE |
Rank |
Pct (%) |
Rank |
Pct (%) |
Rank |
Pct (%) |
Rank |
Pct (%) |
Rank |
Pct (%) |
Rank |
Pct (%) |
Rank |
Pct (%) |
Rank |
Pct (%) |
FOXA1 |
1 |
7.903 |
9 |
3.893 |
1 |
7.927 |
- |
- |
- |
- |
- |
- |
- |
- |
- |
- |
TTN |
2 |
6.586 |
1 |
7.787 |
7 |
4.878 |
- |
- |
- |
- |
4 |
4.545 |
- |
- |
- |
- |
AR |
3 |
6.484 |
3 |
6.148 |
6 |
4.878 |
- |
- |
- |
- |
- |
- |
- |
- |
- |
- |
MUC16 |
4 |
5.876 |
2 |
7.377 |
- |
- |
- |
- |
- |
- |
- |
- |
- |
- |
- |
- |
SPOP |
5 |
5.268 |
11 |
3.893 |
4 |
5.488 |
- |
- |
- |
- |
- |
- |
- |
- |
- |
- |
KMT2C |
6 |
4.661 |
17 |
3.279 |
- |
- |
- |
- |
- |
- |
- |
- |
- |
- |
- |
- |
CDK12 |
7 |
4.458 |
14 |
3.484 |
17 |
3.049 |
- |
- |
- |
- |
- |
- |
- |
- |
- |
- |
MUC4 |
8 |
4.458 |
8 |
3.893 |
- |
- |
- |
- |
- |
- |
1 |
7.955 |
- |
- |
- |
- |
APC |
9 |
4.357 |
4 |
5.738 |
2 |
7.317 |
- |
- |
- |
- |
- |
- |
18 |
4.348 |
- |
- |
CSMD3 |
10 |
4.154 |
18 |
3.074 |
- |
- |
- |
- |
- |
- |
- |
- |
- |
- |
- |
- |
KMT2D |
11 |
4.154 |
7 |
4.098 |
3 |
6.707 |
- |
- |
- |
- |
- |
- |
- |
- |
- |
- |
VPS13B |
12 |
3.647 |
20 |
3.074 |
- |
- |
- |
- |
- |
- |
- |
- |
- |
- |
- |
- |
BRCA2 |
13 |
3.647 |
- |
- |
- |
- |
- |
- |
- |
- |
- |
- |
- |
- |
- |
- |
PTEN |
14 |
3.546 |
5 |
4.918 |
11 |
4.268 |
- |
- |
- |
- |
- |
- |
- |
- |
- |
- |
SYNE1 |
15 |
3.445 |
- |
- |
15 |
3.049 |
- |
- |
- |
- |
- |
- |
- |
- |
- |
- |
MT-CO1 |
16 |
3.141 |
22 |
2.869 |
- |
- |
- |
- |
- |
- |
- |
- |
- |
- |
- |
- |
FAT4 |
17 |
3.04 |
- |
- |
- |
- |
- |
- |
- |
- |
- |
- |
- |
- |
- |
- |
CTNNB1 |
18 |
3.04 |
- |
- |
19 |
3.049 |
- |
- |
- |
- |
- |
- |
- |
- |
- |
- |
RYR2 |
19 |
2.938 |
21 |
2.869 |
- |
- |
- |
- |
- |
- |
- |
- |
- |
- |
- |
- |
KDM6A |
20 |
2.634 |
- |
- |
- |
- |
- |
- |
- |
- |
- |
- |
- |
- |
- |
- |
HMCN1 |
21 |
2.634 |
24 |
2.664 |
- |
- |
- |
- |
- |
- |
- |
- |
- |
- |
- |
- |
ABCA13 |
22 |
2.533 |
- |
- |
- |
- |
- |
- |
- |
- |
11 |
3.409 |
- |
- |
- |
- |
TLE1 |
23 |
2.533 |
- |
- |
- |
- |
- |
- |
- |
- |
- |
- |
- |
- |
- |
- |
RYR3 |
24 |
2.432 |
- |
- |
- |
- |
- |
- |
- |
- |
- |
- |
- |
- |
- |
- |
CSMD1 |
25 |
2.432 |
- |
- |
- |
- |
- |
- |
- |
- |
- |
- |
- |
- |
- |
- |
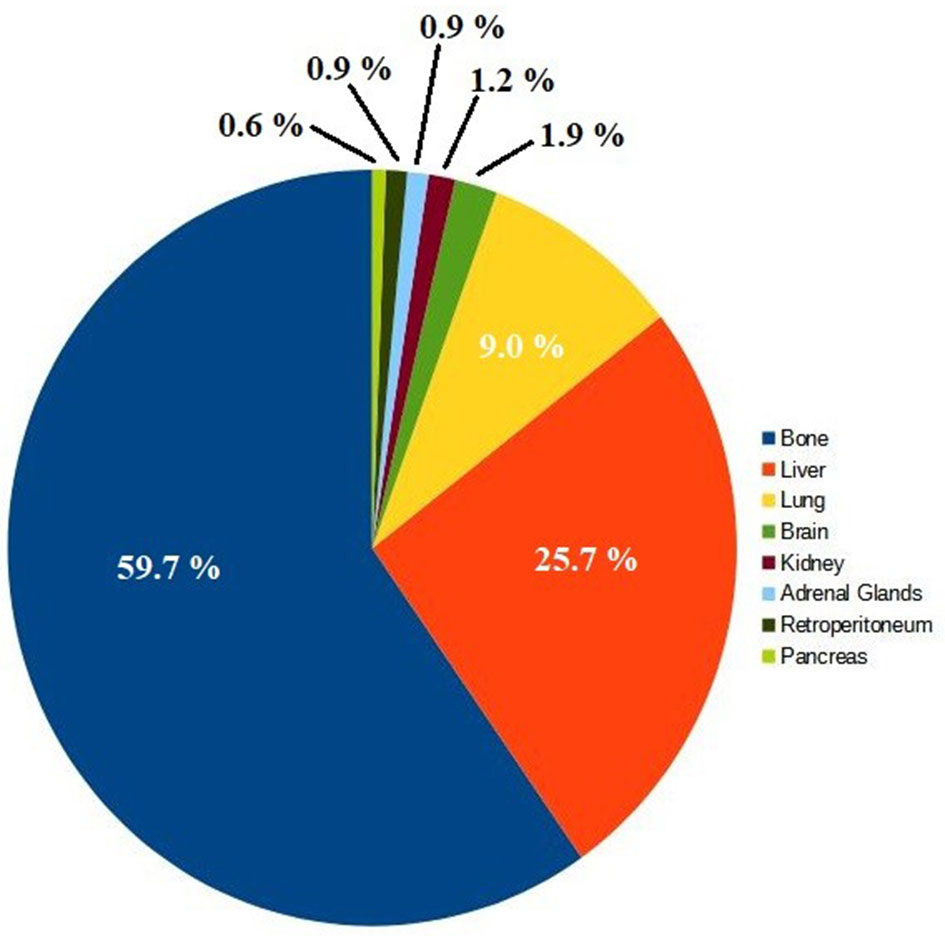
The accuracy was calculated after running the algorithm on all 847 metastatic tumors for which the metastatic site is known. The average accuracy was 80%.
The top three metastatic sites, bone, liver, and lung, are predicted mainly by the large amount of cases and, thus, their large weight in the competition formula to compute the score. The remaining sites, for which a few cases exist, are mainly predicted based on the number of unique genes that are on their uniqueness lists.
Table 4. Prediction of Metastatic Site in Prostate Cancer Accuracy. |
||
Metastatic site |
Cases (n) |
Accuracy |
Bone |
506 |
90.9% |
Liver |
218 |
83.0% |
Lung |
76 |
71.1% |
Brain |
16 |
72.5% |
Kidney |
10 |
80.0% |
Adrenal Gland |
8 |
75.5% |
Retroperitoneum |
8 |
87.5% |
Pancreas |
5 |
80.0% |
This research was aimed at developing a computational tool that could predict a metastatic growth even in the absence of clinical evidence at the time of RP and initial WB-MRI. All metastatic cancers start by shedding circulating tumor cells (CTC) into the circulation. The CTCs that survive the harsh environment of the circulation eventually extravasate to distant organs, giving rise to micrometastases, which in their initial stage cannot be imaged, and thus a false-negative report is presented.
Having a technological tool that warns of the probable presence of metastatic lesions, especially in organs where metastasis is not frequent, serves as an important guide in the treatment of patients with advanced PCa, who, based on the predicted metastases, would be monitored regularly and specifically.
Figure 3 shows an example of the results obtained by entering the mutated genes of a real tumor that metastasized to the lungs. The sample ID on CbioPortal.org is “P-0021894-T02-IM6” and additional information is freely available online on that website. For reference, the complete list of mutated genes is as follows:
CDKN1B, SF3B1, BRD4, FLT4, JAK1, PIK3CD, SPEN, ANKRD11, CSF3R, ERG, KDM5C, KMT2A, PIK3R2, PLCG2, PTPRT, RB1, TET2, ARID1A, CIC, FOXA1, KMT2D, MSH2, NSD1, PAX5, TGFBR2, ANKRD11, BAP1, JAK3, KDM5A, RPS6KA4, APC, CDH1, EPHA3, GRIN2A, KMT2B, KMT2D, NCOR1, RICTOR, INPP4A, IRS2, ZFHX3.
All data generated or analysed during this study are included in this publication.
Acknowledgements
None.
Ethical policy
All procedures performed in this study were in accordance with the ethical standards of the institutional and/or national research committee and with the 1964 Helsinki declaration and its later amendments or comparable ethical standards. Informed consent was obtained from all individual participants included in the study. Approval from institutional ethical committee was taken.
Author contributions
PG: Conception, design of study and manuscript preparation, data collection and analysis and approval for the final version of the manuscript and funding supports.
Competing interests
We declare there are no conflicts of interest.
Funding
No funding was received to support this research.
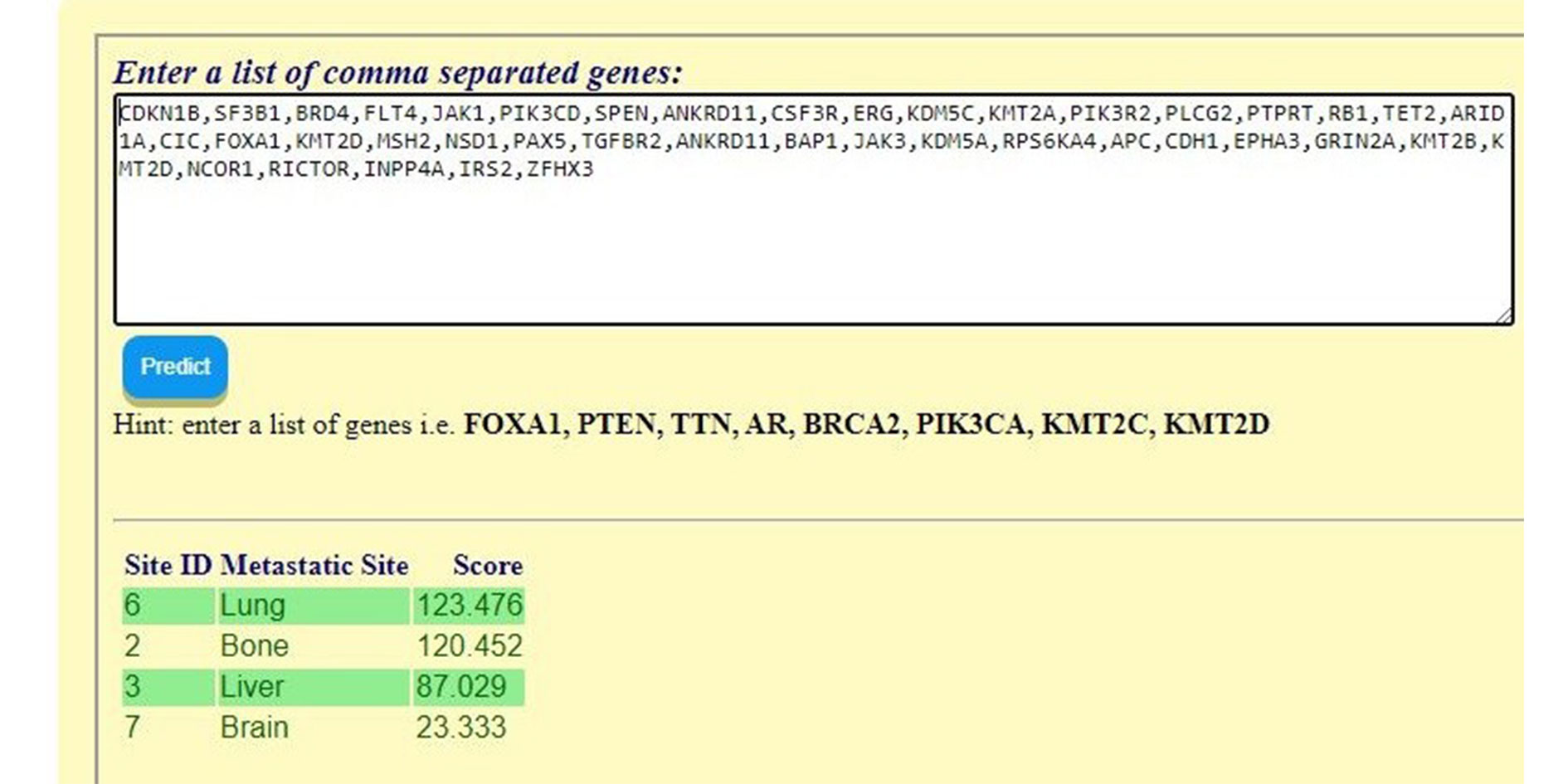
- Quiroz-Munoz M, Izadmehr S, Arumugam D, Wong B et al: Mechanisms of Osteoblastic Bone Metastasis in Prostate Cancer: Role of Prostatic Acid Phosphatase. J Endocr Soc 2019, 3(3): 655-664.
- Manna F, Karkampouna S, Zoni E, De Menna M et al: Metastases in Prostate Cancer. Cold Spring Harb Perspect Med 2019, 9(3): a033688.
- Oliver Sartor, and Johann S: de Bono, Metastatic Prostate Cancer, N Engl J Med 2018, 378(7): 645-657.
- Zhang X, Zhang G, Wang J et al: Different lymph node dissection ranges during radical prostatectomy for patients with prostate cancer: a systematic review and network meta-analysis. World J Surg Onc 2023, 21(1): 80.
- Chen J, Wang Z, Zhao J, Zhu S et al: Pelvic lymph node dissection and its extent on survival benefit in prostate cancer patients with a risk of lymph node invasion >5%: a propensity score matching analysis from SEER database. Sci Rep 2019, 9(1): 17985.
- Thomsen FB, Westerberg M, Garmo H, Robinson D et al: Prediction of metastatic prostate cancer by prostate-specific antigen in combination with T stage and Gleason Grade: Nationwide, population-based register study. PLoS One 2020, 15(1): e0228447.
- Wolff JM, Bares R, Jung PK, Buell U, Jakse G: Prostate-specific antigen as a marker of bone metastasis in patients with prostate cancer. Urol Int 1996, 56(3): 169-173.
- Youmna Kfoury, Ninib Baryawno, Nicolas Severe, Shenglin Mei et al: Human prostate cancer bone metastases have an actionable immunosuppressive microenvironment, Cancer Cell 2021, 39(11):1464-1478.e8.
- Giulia Furesi, Martina Rauner, Lorenz C Hofbauer: Emerging Players in Prostate Cancer–Bone Niche Communication, Trends in Cancer 2021, 7(2): 112-121.
- Liu D, Kuai Y, Zhu R, Zhou C, Tao Y, Han W, Chen Q: Prognosis of prostate cancer and bone metastasis pattern of patients: a SEER-based study and a local hospital based study from China. Sci Rep 2020, 10(1): 9104.
- Nakanishi K, Tanaka J, Nakaya Y et al: Whole-body MRI: detecting bone metastases from prostate cancer. Jpn J Radiol 2022, 40(3): 229-244.
- Zhan Y, Zhang G, Li M, Zhou X: Whole-Body MRI vs. PET/CT for the Detection of Bone Metastases in Patients With Prostate Cancer: A Systematic Review and Meta-Analysis. Front Oncol 2021, 11: 633833.
- Padhani AR, Lecouvet FE, Tunariu N, Koh DM et al: METastasis Reporting and Data System for Prostate Cancer: Practical Guidelines for Acquisition, Interpretation, and Reporting of Whole-body Magnetic Resonance Imaging-based Evaluations of Multiorgan Involvement in Advanced Prostate Cancer. Eur Urol 2017, 71(1): 81-92.
- Cerami E, Gao J, Dogrusoz U, Gross BE et al: The cBio cancer genomics portal: an open platform for exploring multidimensional cancer genomics data. Cancer Discov 2012, 2(5): 401-404.
- Francisco Martínez-Jiménez, Ferran Muiños, Inés Sentís, Jordi Deu-Pons, et al: A compendium of mutational cancer driver genes. Nature Reviews Cancer 2020, 20(10): 555-572.
- Olivier M, Hollstein M, Hainaut P: TP53 mutations in human cancers: origins, consequences, and clinical use. Cold Spring Harb Perspect Biol 2010, 2(1): a001008.
- Marei HE, Althani A, Afifi N et al: p53 signaling in cancer progression and therapy. Cancer Cell Int 2021, 21(1): 703.
- Mantovani F, Collavin L & Del Sal G: Mutant p53 as a guardian of the cancer cell. Cell Death Differ 2019, 26(2): 199-212.
- Heinlein CA, Chang C: Androgen receptor in prostate cancer. Endocr Rev 2004, 25(2): 276-308.
- Formaggio, N., Rubin, M.A. & Theurillat, JP: Loss and revival of androgen receptor signaling in advanced prostate cancer. Oncogene 2021, 40(7): 1205-1216.